|
 |
Mutations occurring in nucleic acids or proteins may affect the binding affinities of protein-nucleic acid interactions. Although many efforts have been devoted to the impact of protein mutations, few computational studies addressed the effect of nucleic acid mutations and explored whether the identical methodology could be applied to the prediction of binding affinity changes upon these two mutation types. Here, we develop a generalized algorithm, named PNBACE, which was capable of effectively predicting the effects of both DNA and protein mutations. We demonstrated that DNA mutations could induce varying degrees of changes in binding affinity from multiple perspectives. We then designed a group of energy-based topological features based on different energy networks, which were combined with our previous partition-based energy features to construct individual prediction models through feature selections. Furthermore, we created an ensemble model by integrating the outputs of individual models using the differential evolution algorithm. Besides the impact of single-point mutations, PNBACE could predict the influence of multiple-point mutations and the mutations significantly reducing binding affinities. Extensive comparisons indicated that PNBACE largely performed better than existing methods on both the regression and classification tasks. |
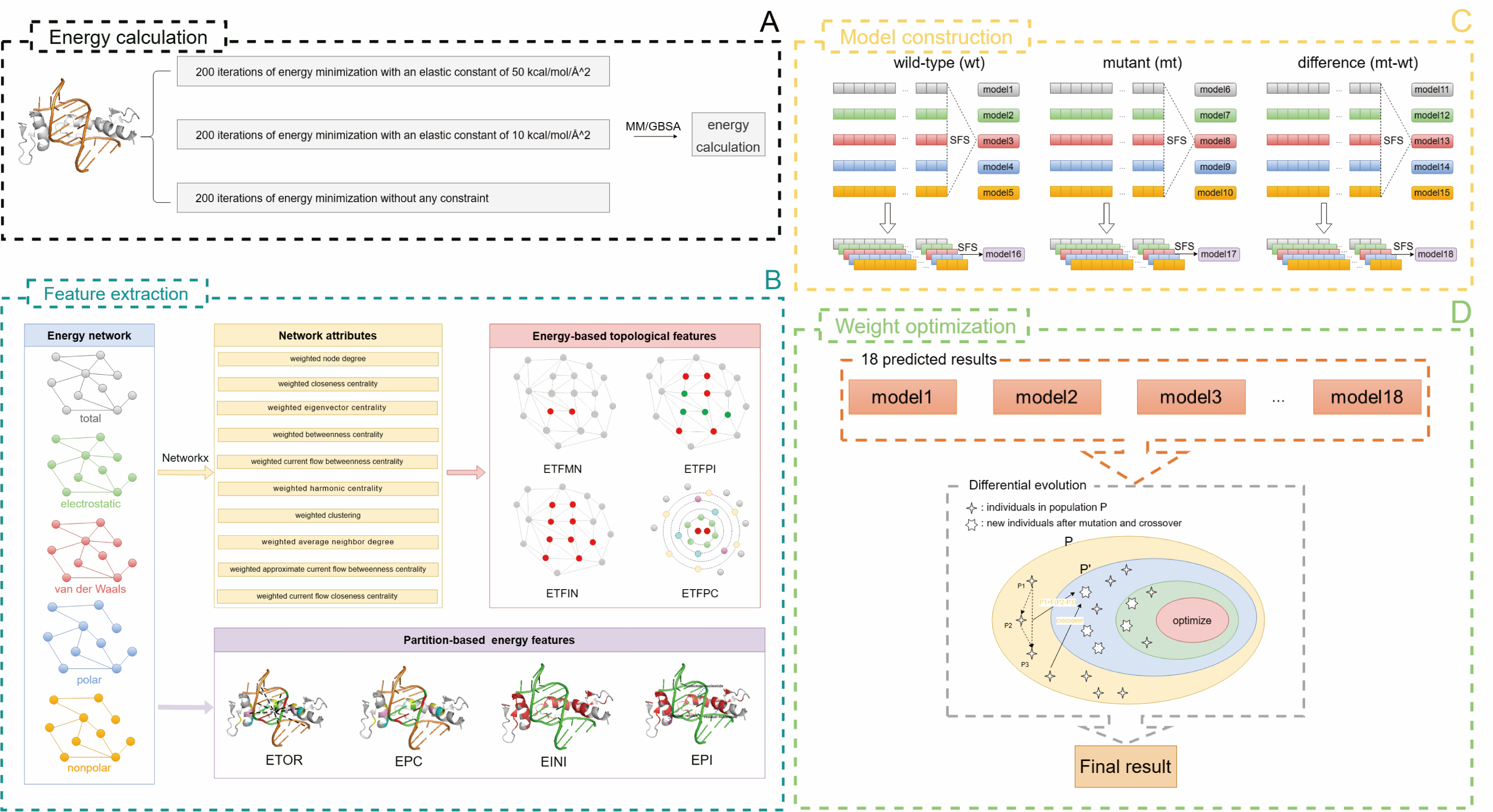
Schematic representation of PNBACE. |
Citation: PNBACE: an ensemble algorithm to predict the effects of mutations on protein-nucleic acid binding affinity. |
|
|
|
|